In today's fast-paced world, the discovery and development of high-tech materials have taken a giant leap forward, thanks to the integration of machine learning and digitalization. This transformative synergy is revolutionizing the way researchers and scientists approach material discovery, offering innovative solutions to complex challenges. In this blog post, we'll explore the impact of machine learning and digitalization on high-tech material discovery through several concrete examples.
Accelerated Screening of Material Properties
Traditionally, identifying materials with specific properties involved laborious and time-consuming experiments. Researchers spent countless hours synthesizing and testing materials to understand their characteristics. However, with the advent of machine learning, scientists can now harness the power of computational modeling to predict material behavior with astonishing accuracy. For instance, consider the field of high-temperature superconductors. These materials, which carry zero electrical resistance, have the potential to revolutionize energy transmission and storage. Through machine learning models trained on vast databases of material properties and structures, researchers can efficiently identify potential superconductors. This expedites the screening process, significantly reducing the time and resources required for material discovery.
Smart Materials for Sustainable Energy
Machine learning and digitalization have ushered in an era of smart materials designed to address our global energy challenges. A prominent example is the optimization of solar panels. Solar energy is a critical component of the sustainable energy landscape, but the efficiency of solar cells was limited by their design and configuration. Machine learning algorithms can analyze a myriad of parameters, such as panel orientation, material composition, and environmental factors, to optimize the energy output of solar cells. Companies like Google's DeepMind have developed algorithms that increase the energy output of solar farms by up to 20%, demonstrating the potential for innovation in renewable energy sources.
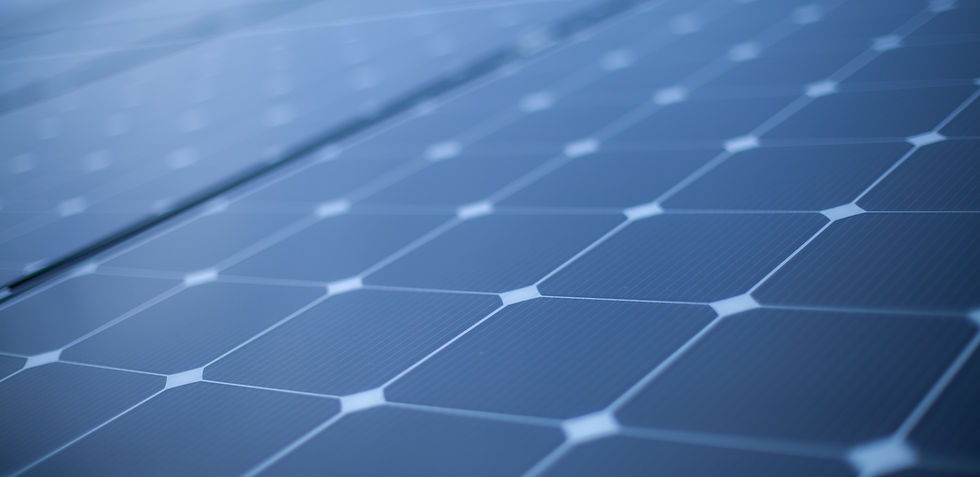
Tailoring Materials for Biomedical Applications
In the field of healthcare, the demand for custom-designed materials has never been greater. These materials are essential for innovations in drug delivery, tissue engineering, and medical devices. Machine learning models play a pivotal role in this endeavor by predicting how different materials will interact with biological systems. Consider the example of drug delivery nanoparticles. Researchers have employed machine learning to design nanoparticles that can precisely target cancer cells while sparing healthy tissue. This not only enhances the efficacy of treatments but also minimizes side effects, showcasing the potential of machine learning in personalized medicine.
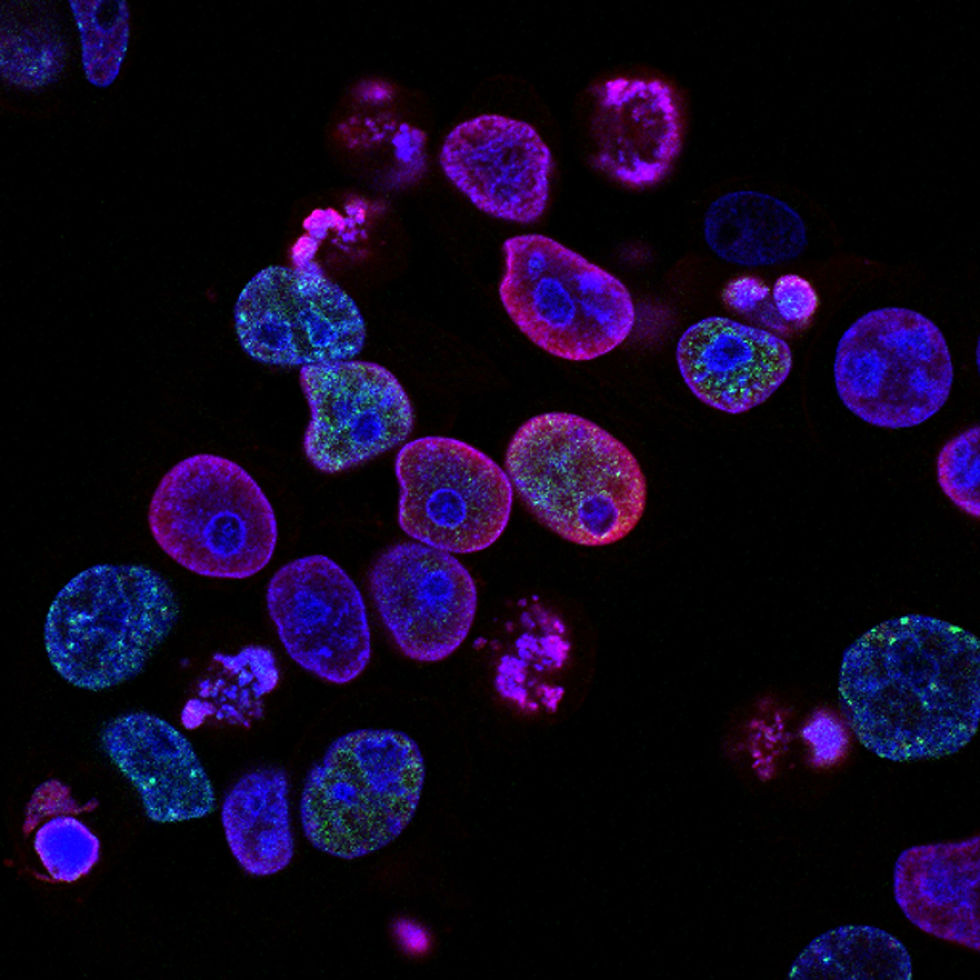
Quantum Materials for Computing
Quantum materials, with their unique properties, hold the promise of supercharging computing power. Machine learning is instrumental in identifying and understanding these materials. Researchers have used machine learning algorithms to sift through vast datasets and discover novel topological insulators, which are essential for the development of quantum computing. This breakthrough could usher in a new era of computing with unparalleled processing capabilities. Quantum computers have the potential to revolutionize industries ranging from cryptography to drug discovery by solving complex problems at speeds that were previously unimaginable.

Sustainable Material Design
The quest for sustainable materials has gained momentum due to growing environmental concerns. Machine learning models are instrumental in evaluating the environmental impact of materials throughout their life cycle. By optimizing materials for recyclability, biodegradability, and eco-friendliness, researchers contribute to a greener and more sustainable future. For instance, the development of biodegradable polymers for packaging materials is a compelling example. These polymers break down naturally, reducing plastic waste and environmental pollution, while still providing the necessary functionality for packaging applications.
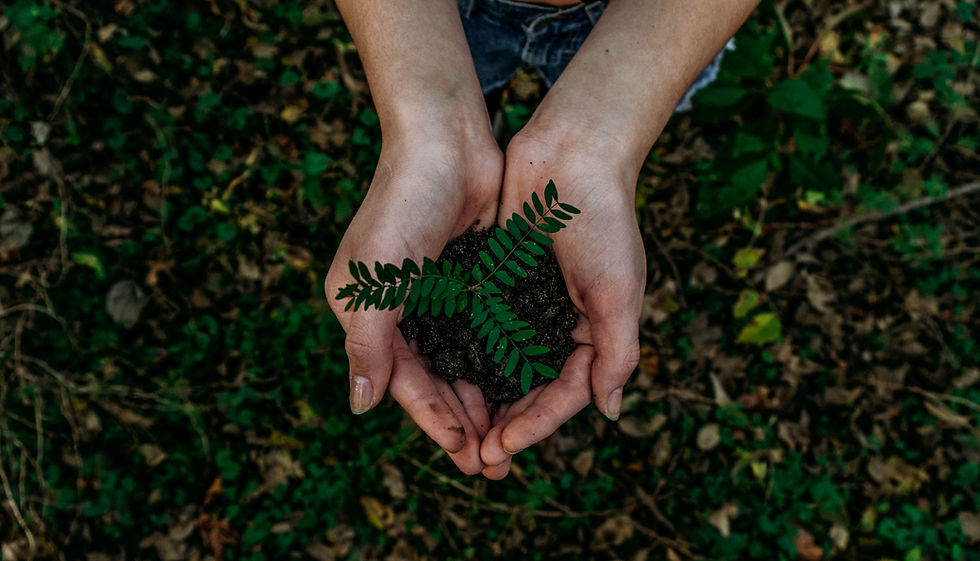
The integration of machine learning and digitalization into high-tech material discovery is a game-changer of monumental proportions. It accelerates the screening of material properties, facilitates the creation of sustainable materials, and accelerates advancements in fields like energy, healthcare, and quantum computing. These innovations not only redefine the limits of material science but also open doors to a more sustainable and technologically advanced future. In a world where technology evolves at breakneck speed, embracing the transformative power of machine learning and digitalization is the key to unlocking the full potential of high-tech material discovery. Researchers and scientists armed with these tools are poised to revolutionize industries, drive innovation, and create a brighter, more sustainable tomorrow. The journey of exploration and discovery continues, guided by the boundless possibilities that machine learning and digitalization offer to the realm of materials science.
Comentarios